Research
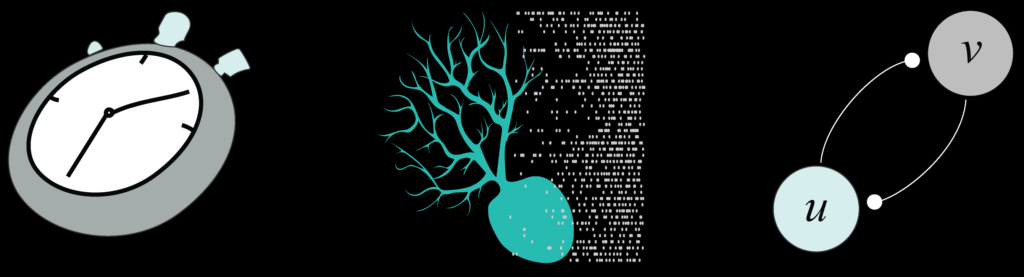
learning under uncertainty
We possess the ability to anticipate and preempt occurrences under familiar circumstances, which suggests a reliance on prior experience with regularities in our environment, especially when observations become more uncertain. This is the key notion behind the study of animal behaviors that are consistent with Bayesian theories. We know little about the neural mechanisms that can learn the probabilities of events in the environment and use this prior experience to guide actions. To examine this, we study rudimentary predictive behaviors in rodents and investigate how prior experience of stimuli create memories that shape behaviors that are statistically optimal.
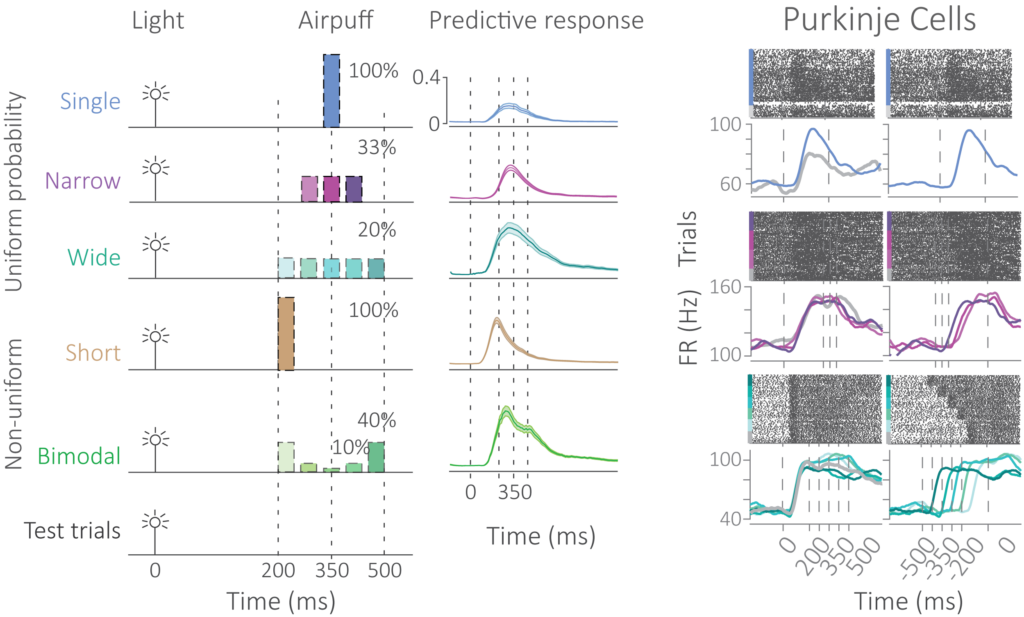
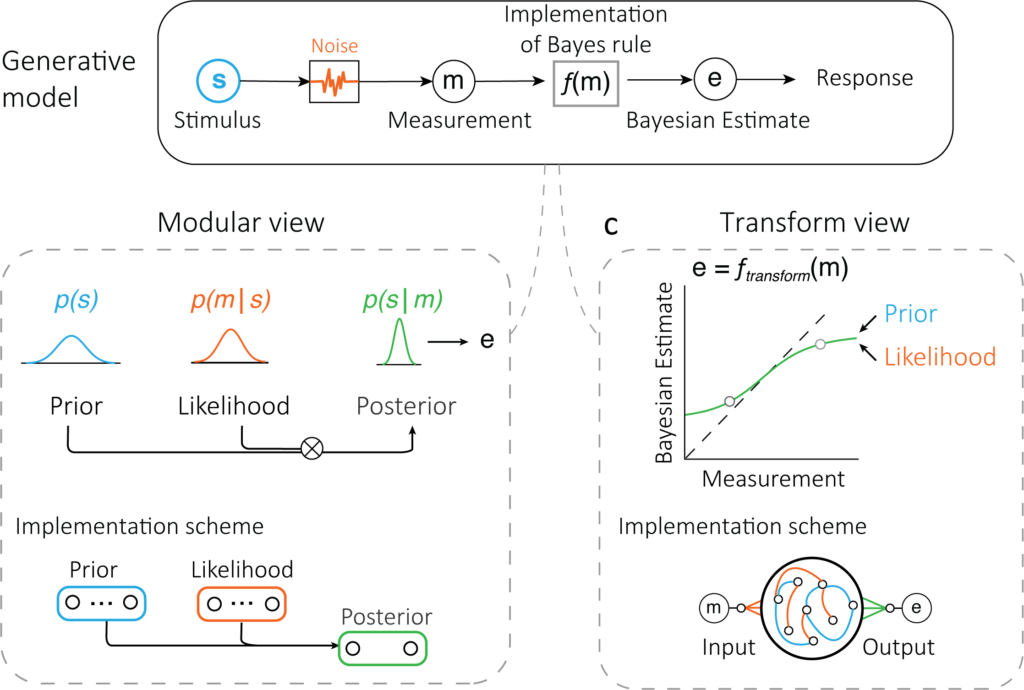
For more information:
- Neural implementations of Bayesian Inference. (2021)
Sohn H and Narain D@
Current Opinion in Neurobiology. 70, 121-129 - Cerebellar encoding of prior knowledge for temporal statistics (under revision)
Koppen J, Runge M, Bayones L, Klinkhamer I & Narain D@
BioRXiv. 2024.08.19.608550
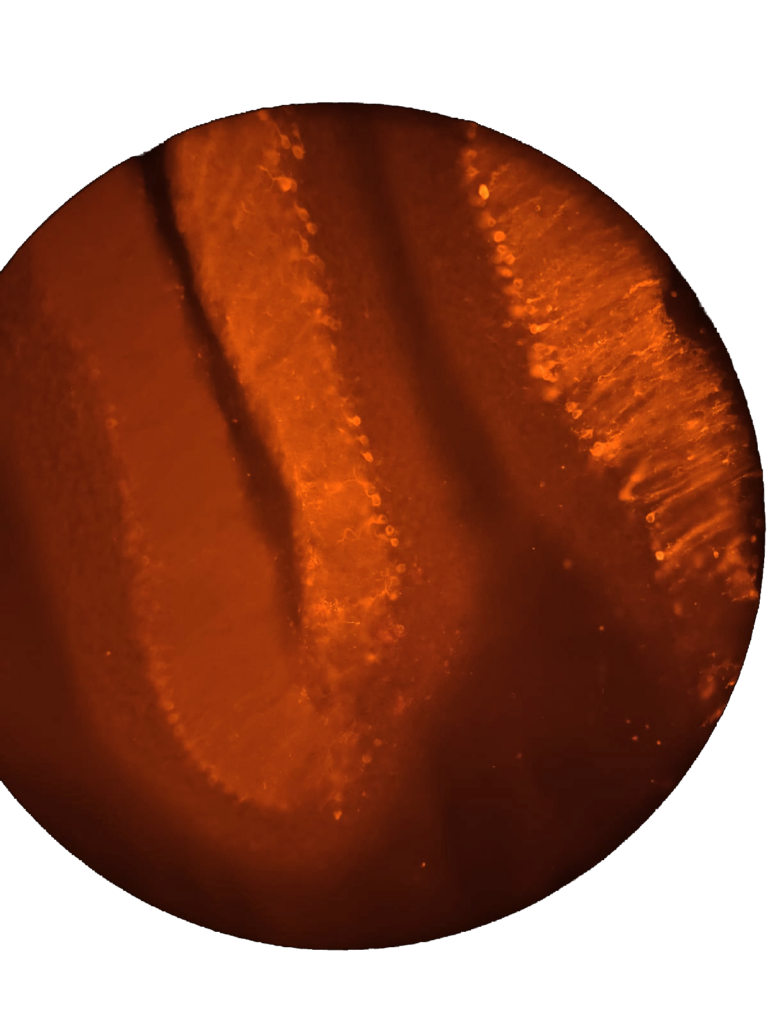
closed loop control to rehabilitate movement
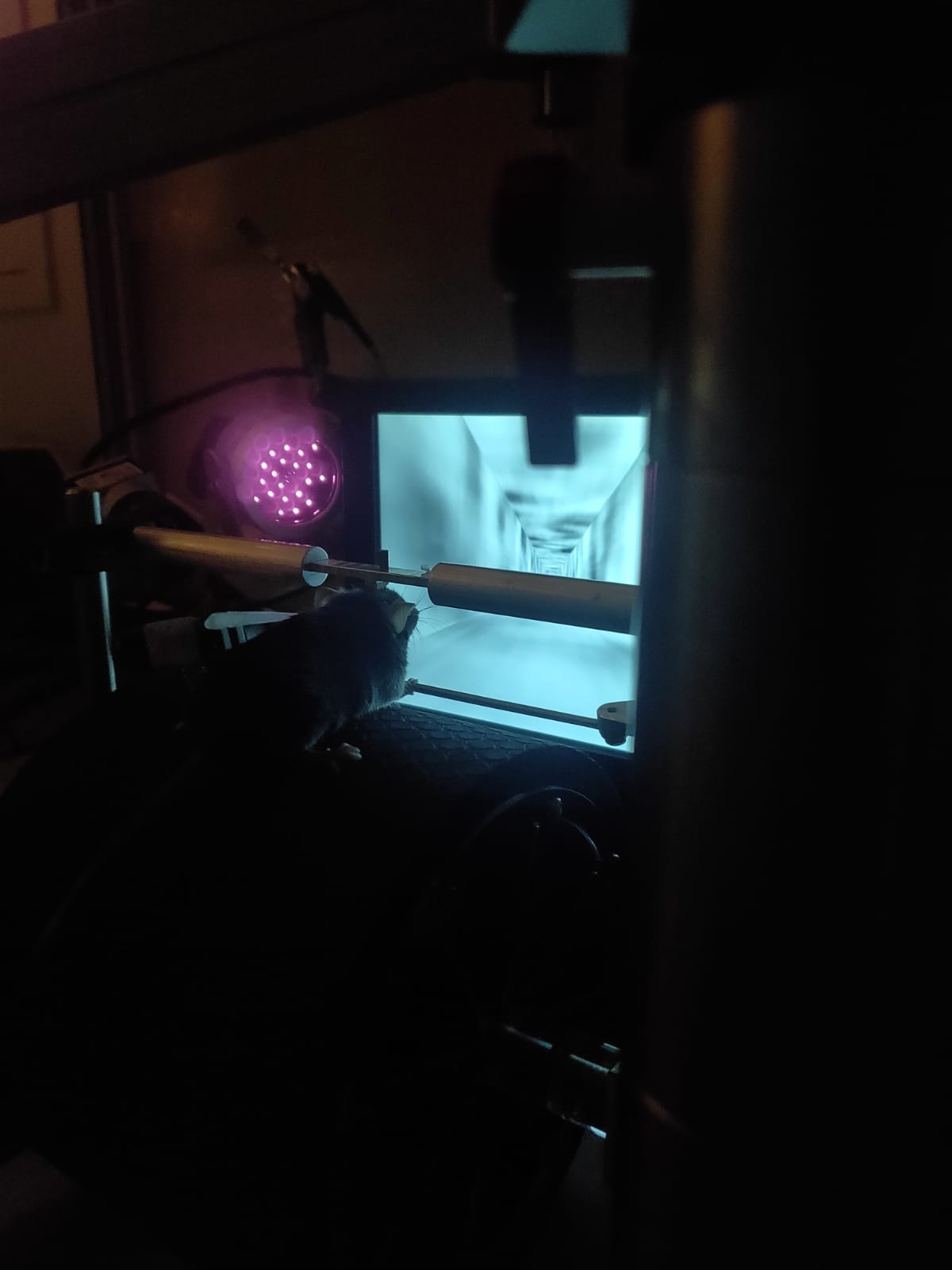
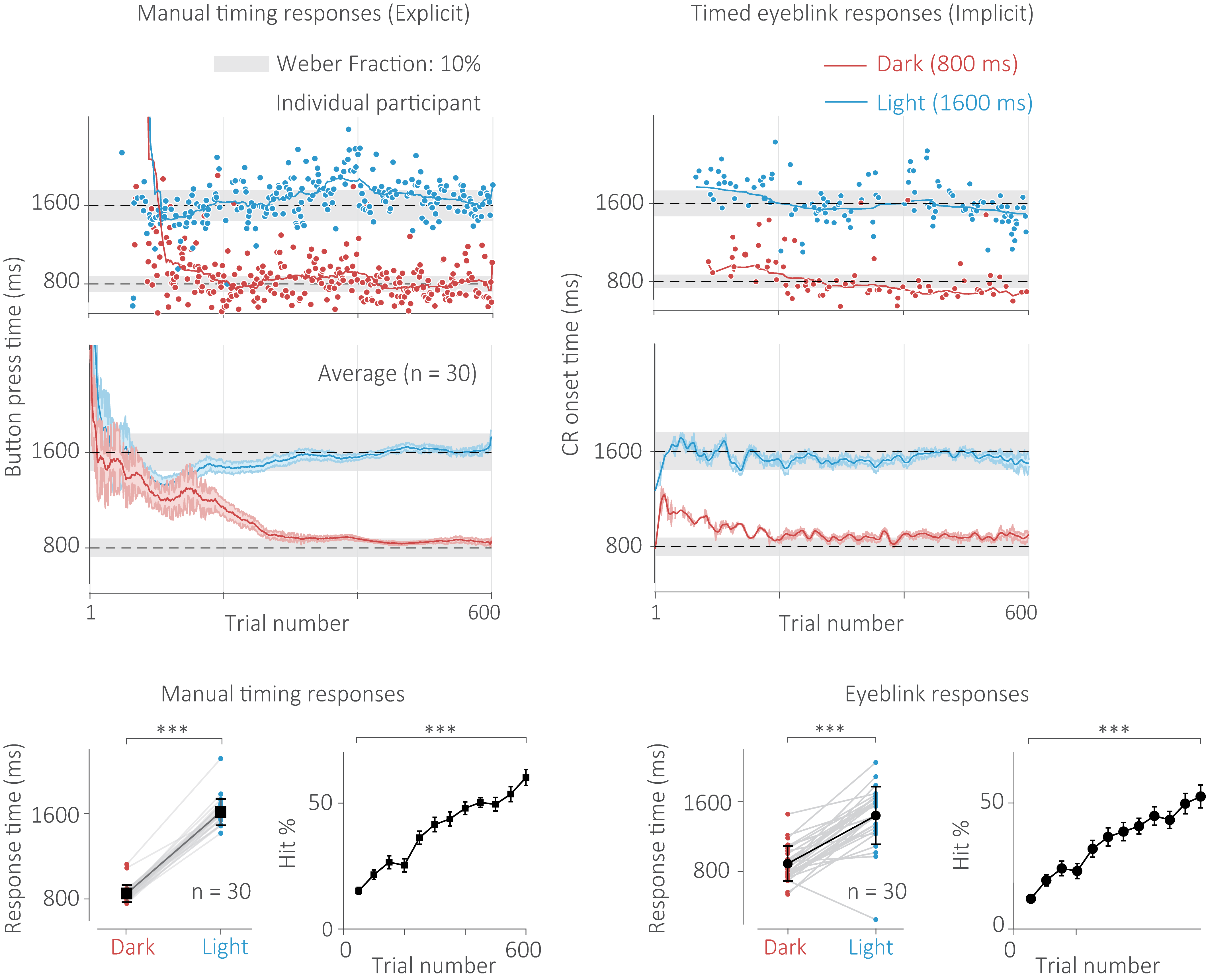
In this line of work, we investigate cortico-cerebellar circuits in in vivo and in silico to understand neural mechanisms behind motor impairment and design control strategies to deliver effective rehabilitative therapy. We examine the interplay between motor learning associated with the cerebral cortex and the cerebellum and study the role that these structures play in generating robust explicit and implicit control of motor behaviors.
For more information:
- Fast implicit and slow explicit learning of motor timing in cortico-cerebellar loops. (2024)
Mangili L*, Wissing C* & Narain D@
BiorXiv. 2024.08.19.608568
Machine learning tools for neuroscience and BCI
In this line of work, we make any methodological developments in our work available to the community. A recent example is RATS: Riemannian Alignment of Tangent Spaces, a bottom-up manifold learning method that generates low-distortion embeddings of high dimensional neural data.
For more information:
- RATS: Low-distortion Riemannian alignment of tangent spaces. (under revision)
Kohli D*, Nieuwenhuis JS*, Cloninger A, Mishne G#@ & Narain D#@
BiorXiv. 2024.05.10.2294108
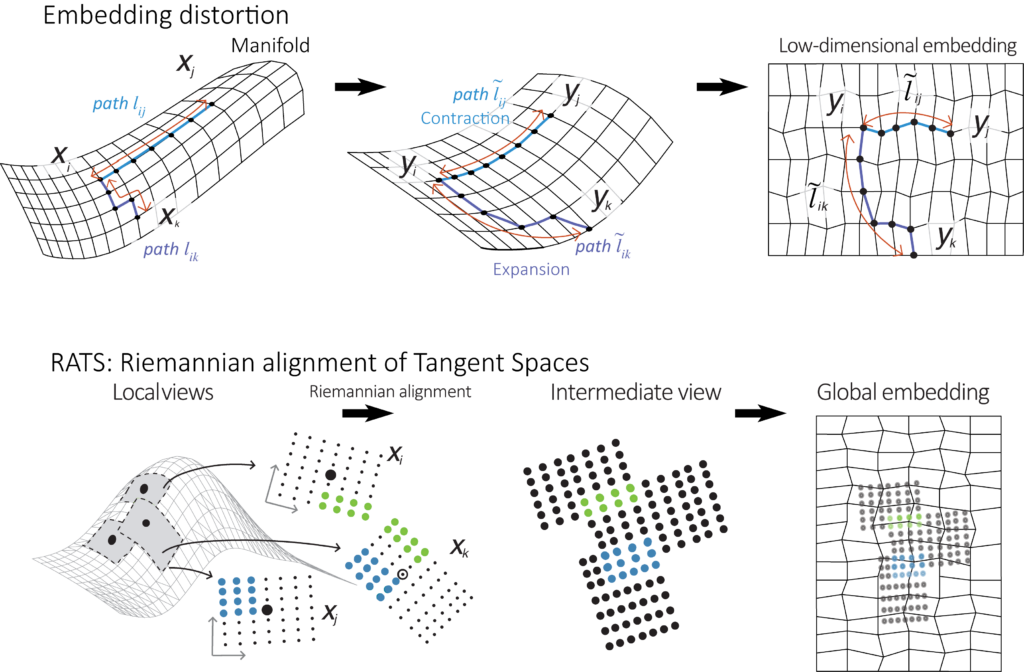